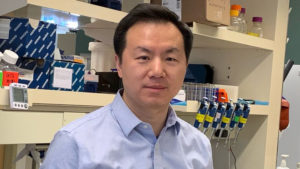
A machine-learning program called Peakachu can reveal previously unknown chromatin loops, structures that are important for gene regulation, according to a Northwestern Medicine study published in Nature Communications.
Developed by a team led by Feng Yue, PhD, the Duane and Susan Burnham Professor of Molecular Medicine, Peakachu employs multiple platforms that search for chromatin loops and uses their combined power to make new discoveries.
“This is the first machine learning strategy to find loops in these genome-wide platforms,” said Yue, also an associate professor of Biochemistry and Molecular Genetics, director of the Center for Advanced Molecular Analysis at the Institute for Artificial Intelligence in Medicine and senior author of the study.
Gene regulation, or controlling which genes are expressed at certain times, is a complex process. Often, regulatory elements such as gene enhancers are positioned far away from the genes that they control.
This necessitates chromatin loops, structures that connect gene regulators to the genes whose expression they modulate. In the case of gene enhancers, this modulation takes the form of enhanced gene transcription, or an increased likelihood that the gene will be activated.
Current methods for identifying chromatin loops include a variety of experimental platforms, all with distinct advantages and limitations. Further, results from different platforms can be quite different, even for the same batch of cells. One reason is that the majority of the current computational approaches mainly look for locally enriched signals in a single platform, according to Yue.
“As each technology has its own limitations, chromatin loops do not always result in a clear pattern that can be readily captured,” said Yue, who is also director of the Center for Cancer Genomics at the Robert H. Lurie Comprehensive Cancer Center of Northwestern University. “This is where machine learning can help boost the detecting power.”
To create a more accurate method, Yue and his collaborators wrote Peakachu, a software program that takes results from many of the currently used platforms, uses a machine-learning algorithm to model and extract the common features, and then uses these features to predict chromatin loops from such genome-wide contact maps.
The group tested Peakachu with over 60 publicly available chromatin interaction data sets in a variety of tissue and cell types, finding that the program not only identified previously discovered loops, but also found a unique set of previously unidentified ones. These newly identified loops tended to be short-range interactions and therefore harder to detect, demonstrating the investigative power of Peakachu.
Another important contribution of Peakachu is that it greatly reduces the need for deep genomic sequencing, according to Yue. While previous methods needed as many as 300 million to 1 billion paired-end sequencing reads to identify high-resolution loops, Peakachu needs just 30 million.
“With our machine-learning strategy, you can just use one-tenth of the reads, and this can be translated in saved cost, which is still a major bottleneck for the study of 3D genome organization,” Yue said. “Further, due to technical challenges, not all biological samples can achieve the desired deep sequencing for detecting high-resolution chromatin interaction. Therefore, this framework and its results could be widely used.”
Identifying these chromatin loops can give scientists a better understanding of how gene regulation works in normal cells, but can also help scientists fight cancer. Many types of cancer have evolved to take advantage of gene enhancers, up-regulating genes that facilitate the growth and proliferation of cancer cells, according to Yue.
“This work can help us identify the enhancers that activate the wrong genes in different types of cancer,” Yue said. “Once we identify what protein binds to the cancer-specific enhancers, it is possible to look for specific inhibitors and drugs for potential targeted therapy.”
This work was supported by National Institutes of Health grants R35GM124820, R01HG009906, U01CA200060 and R24DK106766.