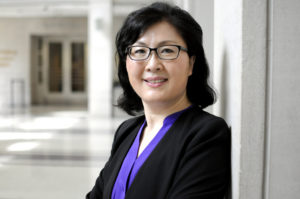
A new machine-learning tool could facilitate population-based epigenetic science by studying the methylation of ‘junk’ DNA, according to a new study published in Clinical Epigenetics.
This new method identified aberrant methylation in these regions, which can help identify hepatitis C-associated liver cancer. The tool will allow scientists to investigate how epigenetic alterations in DNA influence risk of cancer and could lead to biopsy-free diagnostic methods, according to Lifang Hou, MD, PhD, chief of Cancer Epidemiology and Prevention in the Department of Preventive Medicine and director of the Center for Global Oncology at the Institute for Global Health.
“With advances in new technology, many human studies, including several large cohort studies and clinical trials, have generated genome-wide DNA methylation data. But these studies have tended to focus on gene coding regions, with limited attention to DNA repetitive regions due in part to the technical challenges in measuring methylation in these regions,” said Hou, who is also a member of the Robert H. Lurie Comprehensive Cancer Center of Northwestern University. “We are working to overcome this limitation in current technology, and have found that our method does so in a cost-effective manner for population and clinical studies.”
DNA methylation is an epigenetic mechanism that determines whether or not a gene is expressed. Only about two percent of the human genome is made up of genes that code for proteins that have physiological and biological functions, while the remainder is non-coding DNA.
Most of this non-coding DNA is arranged in “repetitive elements,” or stretches of DNA that repeat over and over. Most of the time, these regions are methylated, or turned off, which helps stabilize the genome. However, the field has increasingly recognized that methylation in these repetitive regions exerts some regulatory control over gene expression, especially in aging diseases, according to Hou.
“Are these elements only sleeping? Of course not,” Hou said. “As we get older, methylation in these regions decreases and some of them can activate, leading to human genomic instability and increased mutation rates, thus contributing to cancer and other aging diseases.”
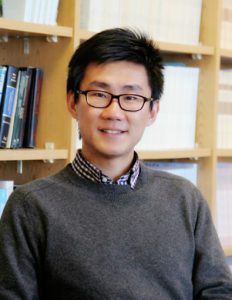
Identifying repetitive elements in DNA and uncovering how their methylation contributes to cancer is an obvious path of inquiry, but it’s hampered by the difficulty of sorting through and tracing thousands and thousands of repetitive and extremely similar DNA sequences. Most tests simply average the amount of methylation throughout these repetitive elements regardless of their genomic origins, losing granularity and detail, said Yinan Zheng, ‘17 PhD, research assistant professor of Preventive Medicine in the Division of Cancer Epidemiology and Prevention and lead author of the study.
“Due to data ambiguities caused by the sequence repetition, current high-throughput technologies have a hard time ‘reading’ the DNA, and thus mostly provide limited coverage on these repetitive regions,” Zheng said. “That’s what we want to look at and improve.”
To do so, Zheng devised a machine-learning tool called REMP. The tool analyzes the relatedness of neighboring sections of DNA and whether or not they’re methylated to estimate DNA methylation in repetitive elements across the human genome. REMP can be applied to DNA methylation data generated by most current platforms, and while the investigators tested this tool with data in cell lines during a previous study, they had not validated REMP in human tissue — until now.
Hou, Zheng and other colleagues studied methylation in liver tissue, over 30,000 repetitive elements in total. Using REMP, the investigators found and validated 15 repetitive elements with aberrant methylation in cancer tissue and 13 of them with significantly lower methylation levels, representing increased genomic instability and a host of other problems consistent with cancer. Similar patterns were also found in hepatitis C-associated cirrhosis, according to the authors.
Because the methylation patterns differed between hepatitis C- and alcohol-induced liver cancers, the investigators were able to further condense these 15 repetitive elements’ methylation into a composite score that could distinguished between hepatitis C and alcoholic hepatitis – two major risk factors of HCC in the U.S. This score could inform treatment, according to Zheng.
“It’s hard to distinguish between these two risk factors when looking at the liver in a clinical setting, but in terms of their effects on liver tissue at the molecular level they are very distinctive,” Zheng said. “The score we created could distinguish between hepatitis C and alcoholic hepatitis, regardless of whether we were looking at liver cancer or liver cirrhosis tissue. In the future, this will have clinical applications by helping to plan treatment accordingly.”
In addition, the authors envision another potential use for this tool: biopsy-free liver cancer diagnoses.
In patients with liver cancer, there are free-floating cancerous cells and cell-free tumor DNA in the bloodstream, a byproduct of the liver’s role in detoxifying blood. As DNA methylation patterns can be tissue-specific, these floating cells or DNA provide important resources not just for research, but also for the early detection of cancer. According to Hou, this tool can thus potentially be used to screen blood samples to evaluate liver cancer risk.
“This could have a huge impact on the early detection of cancers, especially lethal cancers like liver cancer and in countries where cancer screening is not readily available and diagnosis and treatment are prohibitively expensive,” Hou said.
In the future, the authors say they intend to apply REMP to other virus-associated cancers, such as HPV-cervical cancer and HIV-associated cancers, and to continue to pursue clinical and diagnostic uses for the tool.
“These non-coding regions have a complex impact on gene expression and human evolution,” Zheng said. “That’s why understanding these repetitive elements is crucial for understanding the complexity of human diseases. Far from being junk DNA, they may contain priceless information for disease information and treatment.”
This study was supported by the Fogarty International Center of the National Institutes of Health Award Number D43TW009575 and U54CA221205, AASLD Clinical and Translational Research Award in Liver Diseases, and National Institutes of Health grants R01DK110024 and R01AA027179.