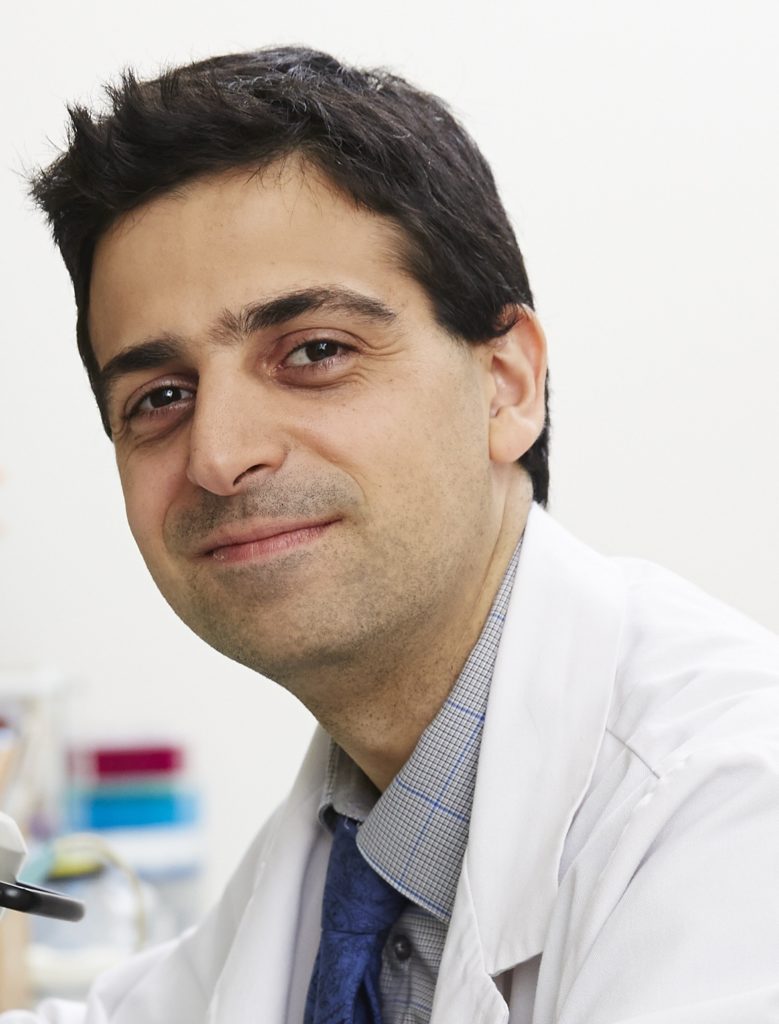
Northwestern Medicine scientists have developed an artificial intelligence (AI) tool that can interpret chest X-rays with accuracy rivaling that of a human radiologist for some conditions, according to findings published in JAMA Network Open.
Jonathan Huang, a student in the Medical Scientist Training Program (MSTP), was the first author of the study.
While the recent boom in AI technology has seen attempts to apply generalist AI models to clinical settings, the lack of medical AI tools built from the ground up inspired Huang to help develop a generative AI model that could assist radiologists in busy emergency departments and provide guidance to physicians working in clinics lacking an on-call radiologist, he said.
“We wanted to leverage our clinical expertise as well as our experience building clinically integrated AI tools to tackle this problem using our own institutional data,” said Huang, who conducted the study in the laboratory of Mozziyar Etemadi, MD, PhD, assistant professor of Anesthesiology and of Biomedical Engineering at the McCormick School of Engineering. “And we built a model that interprets X-rays and complements physician expertise in interpreting medical images by automatically generating text reports from images to help speed clinical workflows and improve efficiency.”
In the study, investigators used 900,000 chest X-rays and radiologist reports to train an AI model to generate a report for each image, describing relevant clinical findings and their significance in the exact same language and style as a radiologist.
Then, investigators had the model interpret 500 chest X-rays taken from an emergency department at Northwestern Medicine and compared the AI reports to those from the radiologists and teleradiologists who originally interpreted the images.
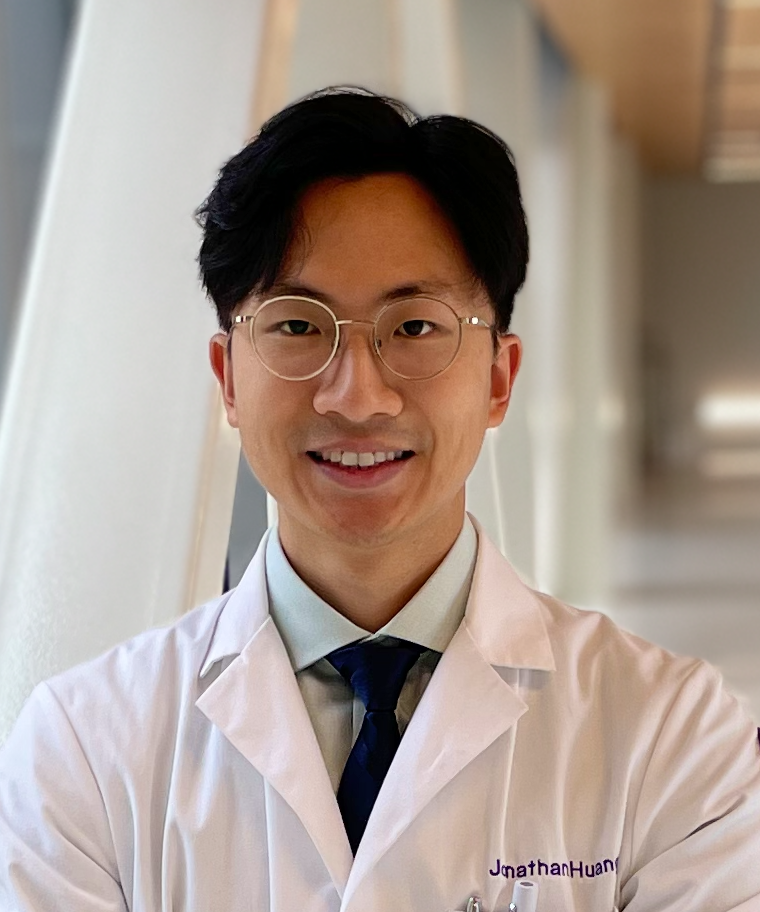
“We wanted to evaluate the AI model’s efficacy in the emergency department setting, which often lacks onsite radiologists who can help advise emergency physicians as they’re seeing patients,” Huang said. “This is a very real clinical use-case where you could imagine an AI model complementing human decision-making.”
Then, five board-certified emergency medicine physicians rated the AI report of each X-ray on a scale of one to five, with five indicating that they agreed with the AI model’s interpretation and no further changes to wording were necessary.
The reviewing physicians found that the AI model was able to accurately flag X-rays with concerning clinical findings and generate a high-quality report on the image, according to the study. Moreover, the study found no difference in accuracy between AI and radiologist reports for all evaluated pathologies, including life-threatening conditions such as pneumothorax and pneumonia.
The study found that the sensitivity and specificity of the AI reports for detecting any abnormality, relative to the on-site radiologists, was 84 and 98 percent, respectively. The original reports from teleradiologists had a sensitivity of 91 percent and specificity of 97 percent for the same task, according to the study.
In a few cases, the AI model was able to identify findings that were missed by human radiologists. In one instance, the AI model identified a pulmonary infiltrate in an X-ray which had not been caught by human radiologists, according to the study.
“When we compared our AI-generated reports to reports that our own in-house radiologists documented, as well as reports that teleradiologists helped provide offsite, we found that our AI reports performed at the same level of clinical accuracy and quality as radiologist reports while providing quality benefits over the teleradiologist reports,” Huang said.
To his knowledge, Etemadi said, this is the first time an AI language model has been used to generate a qualitative report of chest X-rays. Previous studies have used limited AI models to classify image types, but never to holistically interpret medical imagery, he said.
“If you look at AI tools in radiology, they’ve usually been very single purpose, including ones that we’ve previously developed,” Etemadi said. “For example, an AI model that looks at a mammogram and can detect whether or not cancer is present. But in this case, our AI model is telling clinicians everything about an image and giving all the diagnoses, and it can outperform some doctors, basically.”
Building off the initial success with the X-ray AI model, Huang and Etemadi will now work to train the model to read MRIs, ultrasounds and CAT scans, Etemadi said. The AI tool may also be helpful for clinics in areas experiencing a shortage of healthcare professionals and radiologists, Etemadi said.
“We want this to be the radiologist’s best assistant, and hope it takes the drudgery out of their work,” Etemadi said. “We already have started a small pilot study having radiologists evaluate what using this tool in real-time could look like.”
The study was supported internally by Northwestern Medicine.