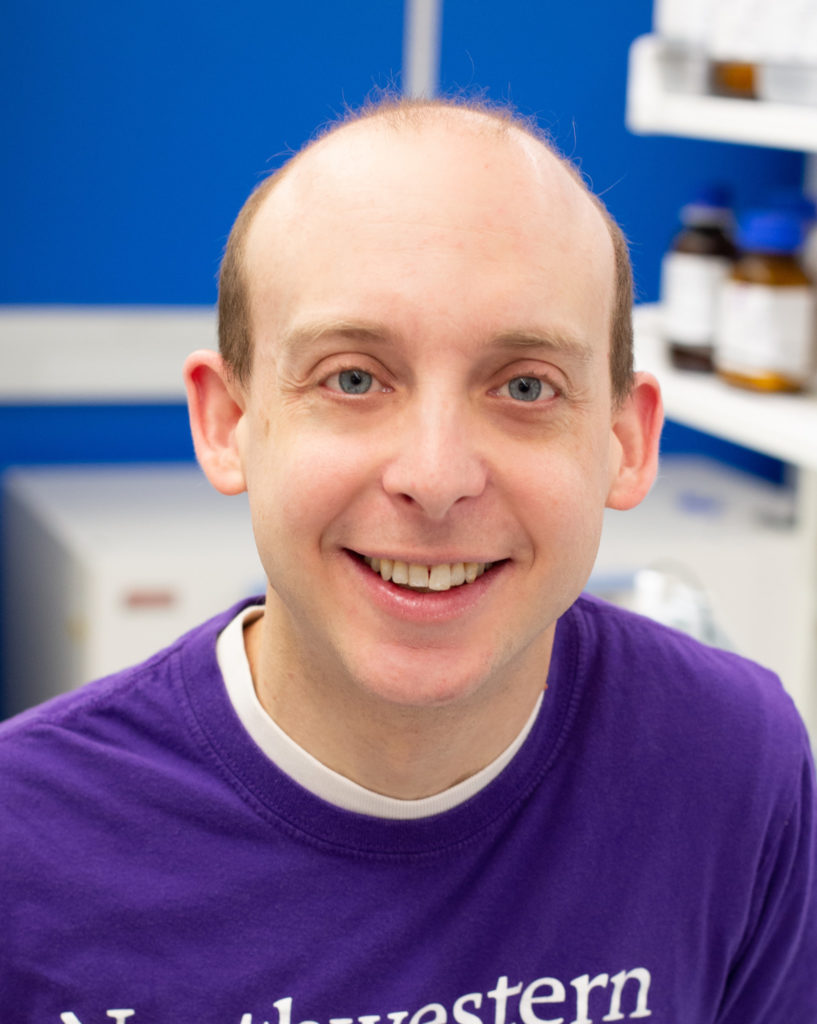
Northwestern Medicine scientists have developed a new technique for measuring protein folding stability on an unprecedented scale, findings detailed in a new study published in Nature.
While advances in technology have helped scientists discover new protein sequences and folded structures, the overall stability of these folded structures are still largely a mystery and new methods are needed to reveal these folding behaviors.
Better understanding of protein folding may provide insight into disease development and protein evolution, as well as guide future approaches to protein engineering and drug development, said Gabriel Rocklin, PhD, assistant professor of Pharmacology and senior author of the study.
“What’s significant about our paper is that we can use this approach to measure folding stability for practically a million different sequences in each experiment,” Rocklin said. “Measuring stability at that scale has always been impossible and a big bottleneck for research. Until recently, the main way scientists measure protein stability is purifying one protein at a time and doing an experiment on that one protein. With individual measurements like this, it’s hard to make predictions about stability for new sequences. By measuring stability on this much larger scale, our data can be used to develop machine learning tools to predict stability and design higher stability proteins.”
In the study, scientists combined the strengths of cell-free molecular biology and next-generation sequencing to develop a new method—dubbed cDNA display proteolysis—to produce a dataset of 776,298 protein folding stability measurements.
Compared to other high-throughput stability assays such as mass spectrometry, the cDNA proteolysis approach produces 100 times more data on protein folding, making it a useful dataset for training machine learning algorithms for experiments involving protein design in the future, according to the authors.
The dataset produced is also unique in that it covers all single protein mutants for 479 different protein domains, as well as more than 200,000 double mutations that provide additional insights into folding. This scale and systematic design make it one of the most comprehensive sources of data of its kind currently available to the scientific community.
“Folding stability is this really important property because it influences function and aggregation and whether a designed protein is going to be a useful therapeutic or not,” Rocklin said. “And if we want to be able to predict that quantity, we need data on this scale. This method that we introduce is the first approach that is going to get us the stability data on the scale that we need for machine learning.”
Moving forward, Rocklin and his collaborators will use the dataset to train machine learning models and use those models for protein engineering, he said.
“What we are working on now is taking the data that we already have and creating a machine learning model with it,” Rocklin said. “We’d also like to take the experimental approach that we have and expand it to all types of protein domains, especially even larger proteins. We’ve already had a lot of interest from other scientists in using our dataset, so we’re excited to empower computational researchers and biophysicists and accelerate machine learning in protein science.”
Kotaro Tsuboyama, a former postdoctoral fellow in the Rocklin lab and currently a lecturer at the University of Tokyo, was lead author of the study.
Rocklin is a member of Northwestern’s Center for Synthetic Biology, Chemistry of Life Processes Institute, and Robert H. Lurie Comprehensive Cancer Center of Northwestern University. The cell-free approaches employed in the study are one of the research specialties of the Center for Synthetic Biology.
The study was supported by Northwestern University Startup Funding, Japan Society for the Promotion of Science grant 19J30003, the Human Frontier Science Program Long-Term Fellowship, and Japan Science and Technology Agency grant JPMJPR21E9.