
Northwestern investigators have developed a deep learning-based method that can predict cognitive function capacity based on brain shape and structure, detailed in a study published in Scientific Reports.
The method, which uses graph convolutional neural networks (gCNNs), may also reveal new insights into the relationship between brain morphology and different cognitive functions as well as the decline of brain function.
“When we apply the rich capabilities of CNNs to graph representation of the brain, we can explore the brain as an image in a previously unexplored way,” said S. Kathleen Bandt, MD, assistant professor of Neurological Surgery and a co-author of the study.
Understanding how the relationship between brain structure and cognitive function changes throughout the life course has remained elusive. However, previous work suggests that fluid intelligence — the ability to problem solve and think and reason abstractly — depends heavily on two regions of the brain: the prefrontal cortex and parietal cortex, both of which are involved in decision-making and sensory perception, among other functions.
Furthermore, investigating the association between brain structure and cognitive function may provide additional information about brain maturation and aging, as well as the physiological causes of cognitive impairment.
In the current study, the investigators developed novel gCNNs, a specialized deep learning model that extracts distinct morphological features such as cortical thickness and subcortical structure from converted brain MRI scans to predict fluid intelligence.
“We are reducing the brain to its surfaces only, meaning that we are able to capture information not only about folding patterns, but also about curvature and relationships between surfaces across tens of thousands of nodes including the outer cortical surface, the inner cortical surface and the surface of seven subcortical brain structures,” Bandt said.
Using their novel gCNNs, the scientists extracted morphologic information of cortical ribbons and subcortical structures from two large MRI datasets involving patients from different age groups.
Using this approach, the investigators were able to demonstrate that their model significantly outperformed other similar state-of-the-art methods and that using a combination of cortical and subcortical structures provided the most accurate predictions.
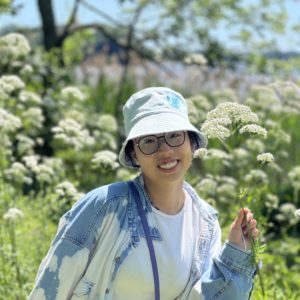
“Prior work investigating the neuroanatomic substrate of fluid intelligence has identified associations between widespread cortical areas, but relatively few relationships have been reported with subcortical structures. Our study added to these studies by identifying involvement of the bilateral NAc, hippocampus in the prediction of fluid intelligence, which have been linked to the aspects of cognitive science such as reward processing in judgment and decision-making as well as emotion regulation,” said Yunan Wu, PhD, a graduate student in the McCormick School of Engineering’s Department of Electrical and Computer Engineering and lead author of the study.
Additionally, across both datasets, they found that structural features of the amygdala, hippocampus and nucleus accumbens (NAc), along with temporal, parietal and cingulate cortex, drove the fluid intelligence prediction.
According to the authors, their surface-based gCNNs offer the opportunity to map identified relationships between neurocognition and brain anatomy for myriad research purposes. The method also requires less training time and computations, making it more efficient to apply to other comprehensive datasets.
For example, another recent study led by Bandt used gCNN analysis for aging and dementia, finding that the pace of brain aging differs between healthy individuals and patients with dementia.
“We are now looking to see if similar cognitive measures can be predicted using this method as has been done in our work here on fluid intelligence, but also can we predict the onset of dementia and potentially prevent or delay it before it starts,” Bandt said.
Co-authors of the study include Pierre Besson, PhD, research assistant professor of Radiology, and Todd Parrish, PhD, professor of Radiology in the Division of Basic and Translational Radiology Research and of Physical Therapy and Human Movement Sciences.