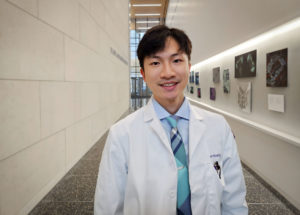
Jonathan Huang, a third-year medical student who grew up in Connecticut, has been fascinated with machine learning (ML) since he was an undergraduate studying computer science at Brown University. Now that Huang is at Feinberg, he is exploring how his burgeoning interest can be applied to the healthcare setting by working with Feinberg scientists, including Mozziyar Etemadi, MD, PhD, assistant professor of Anesthesiology and at the McCormick School of Engineering, who is leading deep learning projects in his lab at Northwestern.
Recently, Huang was the first author of a paper published in JMIR Medical Informatics (of which Etemadi was a co-author and Mahesh Vaidyanathan, MD, MBA, assistant professor of Anesthesiology was corresponding author) called “Evaluation and Mitigation of Racial Bias in Clinical Machine Learning Models.” The study explored best practices for reducing biases in clinical ML research.
Read a Q&A below about this paper and Huang’s other interests, which range from applying ML to vision loss and chest x-ray interpretation, and, in his spare time, checking out Chicago restaurants (Phodega comes highly recommended).
Why did you choose Feinberg?
Listen to Huang below:
I felt immediately welcomed on my interview day here at Feinberg. And since then, in connecting with mentors and fellow classmates, that feeling has been reiterated many times over. I also rely heavily on experiential and active learning, and I knew that curricular elements like ECMH and Problem-Based Learning would help me build my foundation of medical knowledge. Support for research was another important element of my decision, and I knew that I would have ample opportunities to do meaningful work suiting my interests. And of course, Chicago is an incredible place to be, with all there is to experience in food and culture!
What are your research interests?
During my undergraduate studies, which included a degree in computer science, I became enthralled with the seemingly relentless progress that ML has made. Just six years ago, the great ML challenge was a game called Go; today, ML tools can create artwork and stories right in your web browser. The possibilities in medicine are so vast, from workflows that augment medical decision-making to tools that automate away clinical tedium. I’m most interested in developing and implementing these tools, which will let doctors work more efficiently and spend more time with patients.
I’ve been fortunate to work with Dr. Mozzi Etemadi’s team on some of these problems, including a system for flagging potential lung and adrenal nodules identified on radiology reports for further screening, which we built into Epic across Northwestern Medicine and published in The New England Journal of Medicine’s journal, Catalyst. We are also working on validating a system for chest x-ray interpretation — give the model any chest x-ray and it will give you a text report documented just as a radiologist would.
Tell me more about your paper.
Working with Dr. Mahesh Vaidyanathan, co-lead of the digital health curriculum here, our paper was born out of a desire to explore some of the challenges facing clinical ML. Consider real-world issues such as predictive policing or biases in ML-based hiring tools in medicine, where we work daily with uniquely sensitive data, any algorithmic biases could cause patient harm. We’ve seen this in issues such as race-based calculations of kidney function. But while that uses a simple formula with four variables, ML models can have millions of parameters, making them incredibly difficult to interrogate for biases.
In light of this, our paper highlights some challenges and potential solutions to racial bias in clinical ML tools. There has been a lot of recent research done in the algorithmic fairness realm, but these findings take time and awareness to enter common practice. We show that ML best practices for reducing biases are inconsistently applied in clinical ML research, and hope that our paper can be a resource for those seeking to develop and evaluate these tools in clinical settings.
Any next steps for your research?
I’m currently participating in the new Research Intensive Scholarly Emphasis (RISE) program at Feinberg, which provides support for a year of dedicated research. As part of the program, I have been working with Dr. Angelo Tanna and Dr. Mozzi Etemadi on a project to predict progression of glaucoma using optical coherence tomography scans. An ML tool to catch patients at risk of vision loss early in the disease process could help clinicians decide to initiate more intensive treatment regimens for patients who need it most.
What advice would you give to prospective medical students?
Keep an open mind, yet don’t be afraid to commit time to any one specialty or mentor. There are so many different directions to take, whether it be deciding who to shadow, which extracurriculars to pursue, or what research project to take on. Whether or not you end up sticking with it down the road (and there is a lot of road), each experience will help you better define that niche of medicine you would like to make your own.