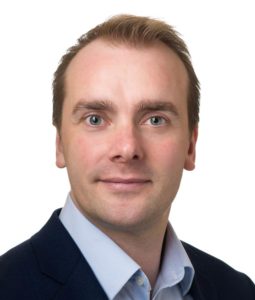
A study co-authored by Northwestern Medicine scientists and published in the journal Cell Stem Cell has shown that analyzing a patient’s own stem cells can predict the safety and efficacy of drugs that have the potential to damage a patient’s heart.
Paul Burridge, PhD, assistant professor of Pharmacology and a member of the Robert H. Lurie Comprehensive Cancer Center of Northwestern University, was a co-author of the study, which investigated using patients’ own stem cells, reprogrammed to develop into cardiomyocytes – heart muscle cells – to test for susceptibility to dangerous side-effects.
“Predicting an individual’s response to a drug is one of the major facets of precision medicine,” said Burridge. “Drug responses in the heart are uniquely difficult to study as we can’t culture heart cells in the lab very efficiently. Stem cell-derived cardiomyocytes are therefore a unique tool for modeling the drug responses of a patient’s heart cells and offer a novel method for the discovery of new drugs and testing for cardiotoxicity in a patient-specific manner. One of our major questions has been how accurate is this model: How much variation does our method of generating cardiomyocytes add in to the system? Do these cells represent the genetic identity of the patient? And can genetic data derived from these cells be used to predict a patient’s drug response?”
Previous research had suggested that various techniques for human-induced pluripotent stem cell (hiPSC) reprogramming may lead to unexpected changes in the new cell’s gene sequence and characteristics. Such variability would call into question tests using the reprogrammed cells, since they would be different from the patient’s cells inside their body.
So the scientists utilized a rigorous bioinformatics approach to investigate any potential variation between reprogrammed cells, which were prepared from five different patients, all of whom were female and had no known genetic mutations associated with cardiovascular disease.
The scientists discovered that when the stem cells were carefully prepared and the process for reprogramming them was standardized, the reprogrammed cells from a single patient were much more similar to other cells from that patient, than cells from any other patient. This similarity indicated that the reprogramming process preserved the specific patient’s unique genetic signature.
Then, to test the reprogrammed stem cells’ ability to predict a patient’s response to therapy, the scientists tested the reprogrammed cardiac cells with the drugs tacrolimus (an immune-suppressant drug) and rosiglitazone (an anti-diabetic drug), both of which act on a protein pathway called the NRF2 pathway. The scientists found that a genetic irregularity in that pathway predicted increased damage to cardiovascular tissues.
The results of this study lend support to the ability of hiPSC to predict clinically-relevant information about an individual patient’s response to certain drugs before they ever receive treatment.
In previous research, Burridge led research showing that reprogrammed stem cells can be used to identify patients with cancer who are likely to experience a dangerous side effect of the common chemotherapy drug doxorubicin.
The new study was supported by National Institutes of Health grants R01 HL113006, R01 HL123968, R01 HL130020, R01 HL126527, and R01 HL128170, Stem Cell Genomics grant (GC1R-06673-A) and NIH grant P01 GM099130, a National Heart, Lung, and Blood Institute Progenitor Cell Biology Jump Start Award, American Heart Association (AHA) grant 16BGIA27790017, a Stanford Cardiovascular Institute Seed Grant (E.M.), NIH grant K99 HL121177 and AHA grant 14BGIA20480329, a Winston Chen Stanford Graduate Fellowship, NIH grant K99 HL104002, AHA grant 15BGIA22730027 and Stanford CVI Seed Grant.