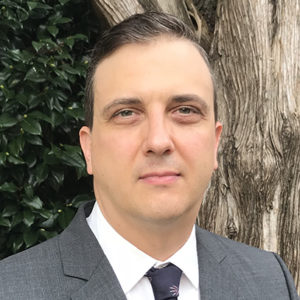
Machine learning could help pathologists more accurately assess how a patient’s immune system is responding to breast cancer, according to a recent series of studies published in the journal NPJ Breast Cancer.
These findings could help direct treatment, and translating the scientific concepts into clinical action is close to reality, according to Lee Cooper, PhD, associate professor of Pathology in the Division of Experimental Pathology and senior author of one review published in NPJ Breast Cancer.
“The research indicates that this technology can work, but we need to validate benefits in clinical trials,” said Cooper, who is a member of the Robert H. Lurie Comprehensive Cancer Center of Northwestern University and director of the Center for Computational Imaging and Signal Analytics in Medicine at the Institute for Artificial Intelligence in Medicine.
When determining the treatment course for patients with breast cancer, one proposed metric is the amount of tumor-infiltrating lymphocytes (TILs) in the tumor — a measure of how responsive a patient’s immune system is to the cancer.
“If immune cells are recognizing and attacking the tumor, one might be able to treat the patient less aggressively and avoid unnecessary negative side-effects,” Cooper said.
Measuring TILs in a reproducible way is challenging, according to a related article published in NPJ Breast Cancer by Cooper and his colleagues. Many pathologists are under significant time-pressure, and automating tasks like TIL quantification with software programs could free up time for interpreting more complex cases.
“These tools can help standardize reporting and reduce variability between pathologists, and can help generalists or community pathologists improve the quality of their diagnoses,” Cooper said.
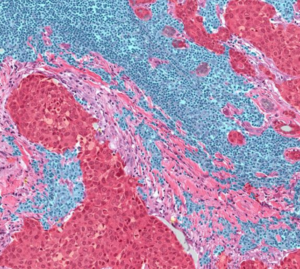
Several groups have developed machine-learning programs to identify and quantify how many TILs are present in a tumor, but cataloguing all parts of the tissue, rather than just TILs, is vital for reproducibility, according to Cooper.
“They have to work this way so they can be validated, so users understand what they’re measuring and how,” Cooper said. “It’s an important step in taking these from research to clinical tools.”
Another critical aspect of the technology is the ability to address pre-analytic factors that influence how the tissues look under the microscope, and how to reconcile these differences across hospital labs.
“Tools that provide standardization would help reproducibility,” Cooper said.
Now, Cooper and his collaborators are working to identify opportunities for including software-based measurements in clinical trials. As with any clinical trial, risk management is an important element, and Cooper and his collaborators offered several strategies in a third article, also published in NPJ Breast Cancer.
Testing pathologists working with the program against those working without is the next step to getting these innovative technologies into a clinical setting.
“People have anxiety that these algorithms will replace experts, but that’s not the case,” Cooper said. “This could help productivity and quality, and make the lives of pathologists easier, in addition to the benefits to patients.”
This study was supported by National Institutes of Health National Cancer Institute (NCI) grants U01CA220401 and U24CA19436201.