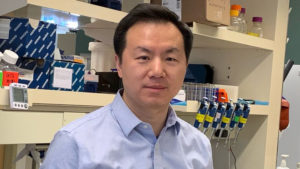
A machine learning model has helped scientists discover hundreds of genetic mutations in cancer that are undetectable by current genome sequencing, according to a study published in the journal Science Advances.
These findings provide new targets for cancer classification and potential therapy, according to Feng Yue, PhD, the Duane and Susan Burnham Professor of Molecular Medicine and senior author of the study.
“Our work identified many previously unknown fusion events in cancer genomes and also captured novel regulatory mechanism for known oncogenes,” said Yue, who is also an associate professor of Biochemistry and Molecular Genetics, of Pathology and director of the Center for Cancer Genomics at the Robert H. Lurie Comprehensive Cancer Center of Northwestern University.
Within each cell, long strands of DNA need to be precisely folded and organized so that they can fit inside the nucleus, which is usually only a few micrometers in diameter. Previously, Yue and his collaborators showed that structural variants in cancer genomes, such as inversions or translocations, can be detected in genomic analytic tools such as Hi-C.
These patterns can be recognized by computer algorithms as indicators for structural variation. Further, these large structural variations are usually missed by whole genome sequencing (WGS) and even long read sequencing such as Nanopore, according to Yue.
“WGS is very good at detecting base pair mutations and short insertions or deletions, but has a hard time detecting larger variation,” said Yue, who is also the director of the Center for Advanced Molecular Analysis at the Institute for Artificial Intelligence in Medicine.
In this study, Yue and his collaborators collected a set of curated high-confidence structural variations of different types from eight cancer cell lines. These were used to train a deep learning model — named EagleC — to learn the hidden patterns buried in these signals. The results were generally concordant with traditional genome sequencing techniques, with 70 to 80 percent of genomic variation also found by either WGS or Nanopore sequencing.
However, EagleC found hundreds of additional fusion events that were missed by whole-genome sequencing or long-reads sequencing. These newly discovered events represent 10 to 20 percent of the total genetic variations detected by Hi-C, according to Yue.
Many of these fusion events cause linkage between an oncogene and a distal enhancer that is usually located on another chromosome. These events, called “enhancer-hijacking,” can lead to upregulation of oncogenes.
In the study, investigators used EagleC to search for structural variation in more than 100 cancer cell lines and patient samples, finding additional fusion events that could be missed by whole genome sequencing. Using this model could expand knowledge of structural variation and their impact on cancer-related genes, according to Yue. This could be especially useful in prostate and breast cancer, two of the most common cancers that also have a high frequency of fusion events.
“We could see if there are differences in therapy response in cancers with fusion events and cancers without,” Yue said. “Our findings also present cancer researchers with many novel regulators that control essential oncogenes and pathways.”
EagleC can also be used to detect structural variation in single-cell Hi-C analysis, where data is sparse. This allows scientists to examine heterogeneity between individual cancer cells.
In the future, Yue said he hopes to apply this model to more cancer samples and look for potential drugs targeting the new fusion events discovered in the current study.
Xiaotao Wang, PhD, postdoctoral fellow in the Yue laboratory, was lead author of the study.
The study was supported by NIH grants 5R01HG011207, 5R35GM124820, 5R01HG009906 and 1U24HG012070.