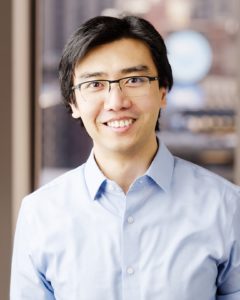
Integrating social determinants of health into machine learning models helped mitigate bias when predicting long-term outcomes for heart failure patients, according to a Northwestern Medicine study published in Circulation: Heart Failure.
The study found that integrating 15 measures of social determinants of health into select machine learning models noticeably reduced disparities observed in predicting the probability of long-term hospitalization or in-hospital mortality for heart failure patients.
“We show that for minority populations, the machine learning models actually performed worse than for white individuals. We also show that for people with poor socioeconomic status, let’s say for those uninsured or for people that have Medicaid, the model also performed worse and missed people that are at a higher risk of dying or have a higher risk of staying in the hospital longer,” said Yuan Luo, PhD, associate professor of Preventive Medicine, of Pediatrics, chief AI officer at the Northwestern Clinical and Translational Sciences (NUCATS) Institute and the Institute for Artificial Intelligence in Medicine, and senior author of the study.
Machine learning can be a powerful tool for predicting long-term patient outcomes, especially for diagnosed with chronic conditions such as heart failure. While models can improve the dissemination of health resources during patients’ course of care and overall health outcomes, the fairness of such models had yet to be thoroughly investigated.
In the current study, investigators studied the performance of five machine learning models commonly used to determine the probability of length-of-stay and mortality in hospitalized patients.
Into these models, the investigators integrated 15 social determinants of health provided by the American Heart Association. These variables included Social Deprivation Index scores, which are based on seven demographic characteristics to quantify an area’s socio-economic variation in health outcomes, and Area Deprivation Index scores, which rank different areas by socioeconomic disadvantage based on factors including income, education, employment and housing quality.
Overall, they found that the best-performing model initially underdiagnosed several underserved patient populations, including female, Black, and socioeconomically disadvantaged patients. However, integrating social determinants of health improved the fairness of the models without compromising their performance.
“In contrast with conventional attitudes like, ‘you need to make a tradeoff,’ we show that you actually don’t need to make the tradeoff. We can keep the same levels of utility while increasing fairness,” said Luo, who is also a member of the Robert H. Lurie Comprehensive Cancer Center of Northwestern University
Machine learning models are often affected by the biases in the utilized data, Luo said, but the extent to which these biases existed in the current models was surprising, adding that the current study provides a framework for investigators to develop high-performing predictive models for patients that also reduce disparities.
“Our purpose is to also let people know that you can actually correct these models by adding those social determinants of health variables, making models explicitly aware of such variables so that they can learn to correct for those biases,” Luo said.