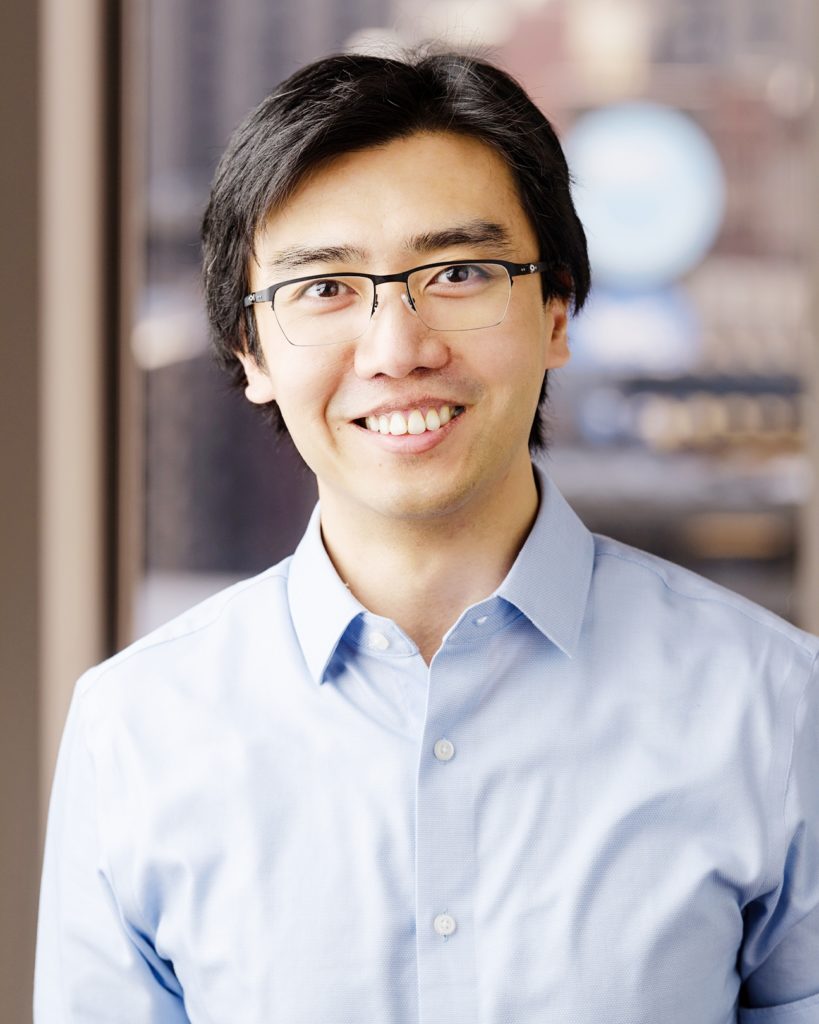
An AI model developed by Northwestern Medicine investigators improved the transformation of EHR data into standardized health resources more efficiently than current methods, according to a recent study published in the journal NEJM AI.
The model, FHIR-GPT, uses the power of large language models to convert clinical data into Fast Healthcare Interoperability Resources (FHIR) resources, and is a step towards advancing health data interoperability, research, clinical trial support and public health surveillance, according to Yuan Luo, PhD, associate professor of Preventive Medicine in the Division of Health and Biomedical Informatics, director of the Center for Collaborative AI in Healthcare and senior author of the study.
“This is going to greatly accelerate the pace of breaking down the walls between different health systems that hinder the aggregation of health data and the exchange of the data for performing large-scale research, which we are in great need of, especially with the advancement of the generative AI and large language model technology,” said Luo, who is also a professor of Pediatrics, chief AI officer at the Northwestern University Clinical and Translational Sciences (NUCATS) Institute and the Institute for Artificial Intelligence in Medicine, and a member of the Robert H. Lurie Comprehensive Cancer Center of Northwestern University.
Health data interoperability is essential for improving patient care, but also for achieving health equity to responding to public health emergencies, according to the authors.
To accelerate this essential data exchange, U.S. federal agencies, including the Office of the National Coordinator of Health Information Technology, the Centers for Disease Control and Prevention, and the Centers for Medicare & Medicaid Services facilitated the adoption of the Fast Healthcare Interoperability Resources (FHIR) standard.

Initially developed in 2011 by the international standards organization Health Level 7, FHIR is a set standard for exchanging electronic health records (EHRs) between health organizations, including academic medical centers and commercial industries, to ensure efficient exchange of clinical and administrative information regardless of how it is stored.
FHIR also supports different health research applications, including computational phenotyping, clinical trial support, and the development of surveillance systems.
“FHIR is like a universal language for healthcare data, much like English is for international communication. When hospitals adopt FHIR, they can seamlessly share and understand each other’s data, leading to better collaboration and improved patient care,” Luo said.
Because health organizations have their own unique infrastructures, standards and formats for generating, storing and organizing health data, transforming health data into FHIR resources is challenging, according to Luo.
“Healthcare in the U.S. is highly fragmented,” Luo said. “Each hospital uses its own customized version of EHR systems, creating data that is often bespoke and difficult to exchange and interpret due to the nuances in templates and formats. FHIR aims to bridge these gaps by providing a common language for data exchange, enabling hospitals to seamlessly share and aggregate information. This standardization helps overcome the barriers of fragmentation, improving communication and collaboration across the healthcare system.”
In the current study, Luo’s team developed an FHIR-trained large language model that converts EHR data into FHIR medication statements and compared their model’s performance to current state-of-the-art systems. More than 3,600 pieces of clinical text were manually annotated by Luo’s team and then used to prompt GPT-4, Open AI’s large language model.
From these experiments, FHIR-GPT perfectly matched EHR data into FHIR medication statements with a 90 percent success rate, surpassing the performance of existing tools.
FHIR-GPT also improved exact match rates of these tools by 3 percent for medication administration routes, 12 percent for dose quantities, 35 percent for reasons for medication administration, 42 percent for medical forms, and more than 50 percent for medication timing schedules.
The system is not only more accurate than other systems but is also more cost-efficient to develop and more scalable, according to the authors.
“With the help of large language models, we can standardize the data into a standardized format so then we can build a large data set and it is easy to communicate with different stakeholders in healthcare,” said Yikuan Li, MS, a fifth-year student in the Health Sciences Integrated PhD Program and lead author of the study.
Luo said his team aims to validate the system further and eventually deploy it within existing healthcare systems across the U.S. to advance the aggregation of health data from diverse patient populations and improve patient care and health equity.
“If you think about what kind of research or insights could come up with this better integrated, much larger and diverse cohort, the opportunity is going to be tremendous,” Luo said.
This work was supported by the National Institutes of Health grants R01LM013337 and U01TR003528 and by an American Heart Association Predoctoral Fellowship (grant 23PRE1010660).