MRI scans will allow intervention with therapy to maximize language learning
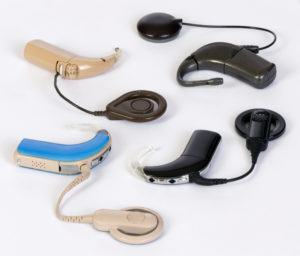
Scientists created a machine learning algorithm that uses brain scans to predict language ability in deaf children after they receive a cochlear implant, allowing clinicians to maximize language learning for the child.
The study, published in the Proceedings of the National Academy of Sciences, was a collaboration between Ann & Robert H. Lurie Children’s Hospital of Chicago, Northwestern University Feinberg School of Medicine and the Chinese University of Hong Kong.
“The ability to predict language development is important because it allows clinicians and educators to intervene with therapy to maximize language learning for the child,” said co-senior author Patrick C. M. Wong, PhD, a cognitive neuroscientist, professor and director of the Brain and Mind Institute at The Chinese University of Hong Kong. “Since the brain underlies all human ability, the methods we have applied to children with hearing loss could have widespread use in predicting function and improving the lives of children with a broad range of disabilities.”
A cochlear implant is the most effective treatment for children born with significant hearing loss when hearing aids are not enough for the child to develop age appropriate listening and language ability. Decades of studies have shown that early cochlear implantation is critical.
About 38,000 cochlear implants have been implanted in children in the U.S. as of December 2012, according to the National Institute on Deafness and Other Communication Disorders.
Although a cochlear implant enables many children with hearing loss to understand and develop speech, some children lag behind their normal hearing peers despite receiving an implant as an infant or toddler. Helping these children achieve the language and literacy of hearing children is important and the focus of much investigation, as these skills are critical to academic success, social and emotional well-being, and employment opportunities.
“So far, we have not had a reliable way to predict which children are at risk to develop poorer language,” said co-senior author Nancy Young, MD, ’87 GME, medical director of Audiology and Cochlear Implant Programs at Lurie Children’s and professor of Otolaryngology. “Our study is the first to provide clinicians and caregivers with concrete information about how much language improvement can be expected given the child’s brain development immediately before surgery. The ability to forecast children at risk is the critical first step to improving their outcome. It will lay the groundwork for future development and testing of customized therapies.”
This study’s novel use of artificial intelligence to understand brain structure underlying language development has broad-reaching implications for children with developmental challenges.
“A one-size-fits-all intensive therapy approach is impractical and may not adequately address the needs of those children most at risk to fall behind,” Wong said.
Successful hearing and spoken language development depends on both the ear and the brain. Hearing loss early in life deprives the auditory areas of the brain of stimulation, which causes abnormal patterns of brain development.
Erin Ingvalson, PhD, assistant professor at Florida State University who began work on the project as a postdoctoral student at Northwestern University, said “our goal is to eliminate the gap in language outcomes often found when children with hearing loss are compared to those with normal hearing. The ability to optimize therapy for each child with hearing loss will transform many lives.”
“We used MRI to capture these abnormal patterns before cochlear implant surgery and constructed a machine-learning algorithm for predicting language development with a relatively high degree of accuracy, specificity and sensitivity,” Wong explained. “Although the current algorithm is built for children with hearing impairment, research is being conducted to also predict language development in other pediatric populations.”